The world of Artificial Intelligence (AI) is abuzz with excitement. Cutting-edge models are pushing the boundaries of what’s possible, from generating lifelike images to predicting complex human behavior. However, the journey from powerful models to practical applications often gets overlooked. The missing piece in this puzzle? The foundational stack – a robust infrastructure that bridges the gap between theoretical models and real-world impact.
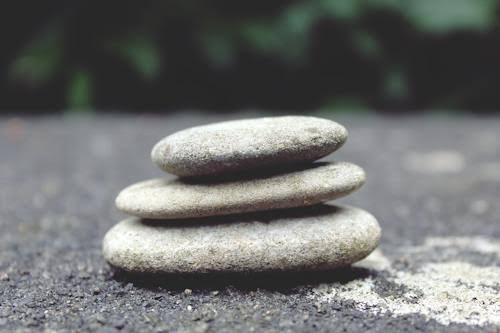
Why is the Foundational Stack Crucial?
A recent study by source: IDC, The Global Artificial Intelligence Spending Guide, 2024 predicts that global spending on AI will reach $576.6 billion by 2025. This surge in investment highlights the immense potential of AI, but it also underscores the need for a strong foundation to realize this potential.
Here’s why the foundational stack is critical:
Scalability and Efficiency: Powerful AI models often deal with massive datasets and complex computations. The foundational stack ensures the infrastructure can handle this workload efficiently and at scale.
Security and Reliability: AI applications often handle sensitive data. The foundational stack needs robust security measures to protect data integrity and ensure reliable system operation.
Integration and Deployment: The stack should seamlessly integrate with existing systems and enable smooth deployment of AI models into real-world applications.
Explainability and Transparency: As AI becomes more pervasive, explainability of models becomes crucial. The ideal foundational stack can accommodate explainability tools and techniques.
Beyond AI/ML Expertise: The Skills Needed
While AI and machine learning expertise are crucial, building a robust foundational stack requires a broader skillset:
- Foundational Engineering: Solid understanding of software development principles, system architecture, and data engineering practices.
- System Design: The ability to design scalable and secure systems capable of handling complex data flows and computations.
- Application Engineering: Knowledge of building real-world applications that integrate seamlessly with AI models.
- DevOps and MLOps Practices: Integration of DevOps and MLOps practices ensures efficient and reliable development, deployment, and monitoring of the foundational stack and AI models.
Ovonts: Building a Foundation for Explainable and Collaborative AI
At Ovonts, we believe in a holistic approach to AI development. Our suite of tools empowers developers to build explainable and collaborative AI models. This aligns perfectly with the concept of a strong foundational stack.
By promoting context-awareness, explainability and collaboration, Ovonts contributes to creating a robust and user-centric foundational stack for AI. This promotes trust and transparency in AI decision-making, fostering wider adoption and responsible use of this powerful technology.
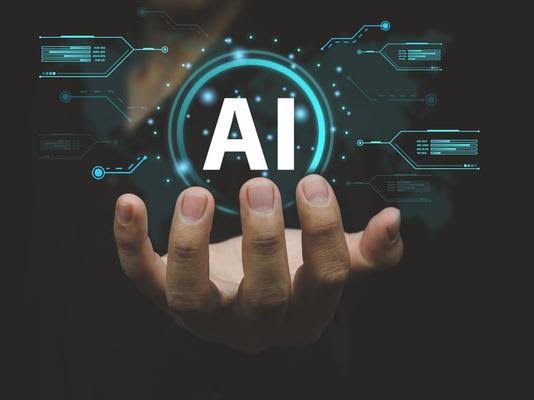
The Road Ahead: Building the Future with a Strong Foundation
As AI continues to evolve, the importance of the foundational stack will only increase. By focusing on building a solid infrastructure, organizations can bridge the gap between theoretical models and real-world applications. This paves the way for groundbreaking possibilities across various industries:
Healthcare: Improved diagnostics, personalized medicine, and efficient drug discovery.
Finance: Risk management, fraud detection, and automated financial analysis.
Manufacturing: Predictive maintenance, optimized supply chain management, and intelligent robotics.
Retail: Personalized recommendations, enhanced customer experiences, and demand forecasting.
Government and Defence agencies: National security, decision making and transformations.
By investing in the foundational stack and collaborating with tools like Ovonts that prioritize context -awareness, explainability and collaboration, we can unlock the true potential of AI and build a more responsible and innovative future.